Quantum chemistry
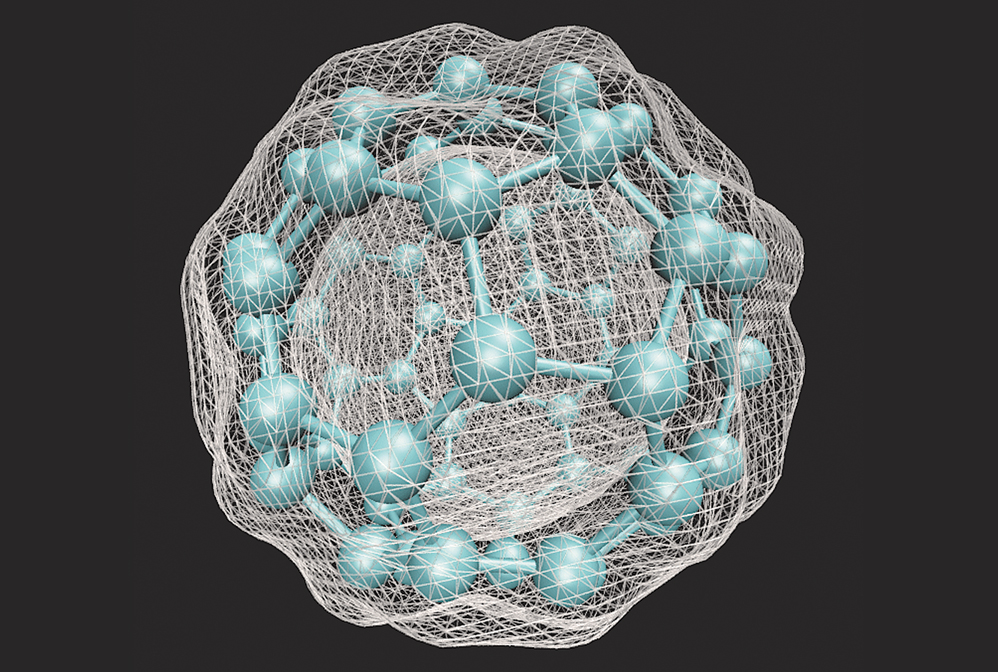
Density functional theory calculations allow chemists to predict physical properties of molecular materials such as the electron density of C60.
Credit: Itamblyn/Wikimedia Commons
Chemists began applying the principles of quantum mechanics to the study of molecular systems nearly a century ago. Yet in the late 1920s, when Erwin Schrödinger published his namesake equation, there were no computers powerful enough to solve the wave function for whole molecules. Quantum chemistry calculations soon became the foundation of how scientists understood the nature of matter. As computer scientists introduced new ways to program computers to execute complex commands, chemists developed methods to generate chemical systems from first principles in addition to data from experiments. One such method, called density functional theory, is used for calculating electron density and distribution in molecular systems and has become one of the most powerful and pervasive quantum mechanical modeling tools in chemistry.
Drug discovery
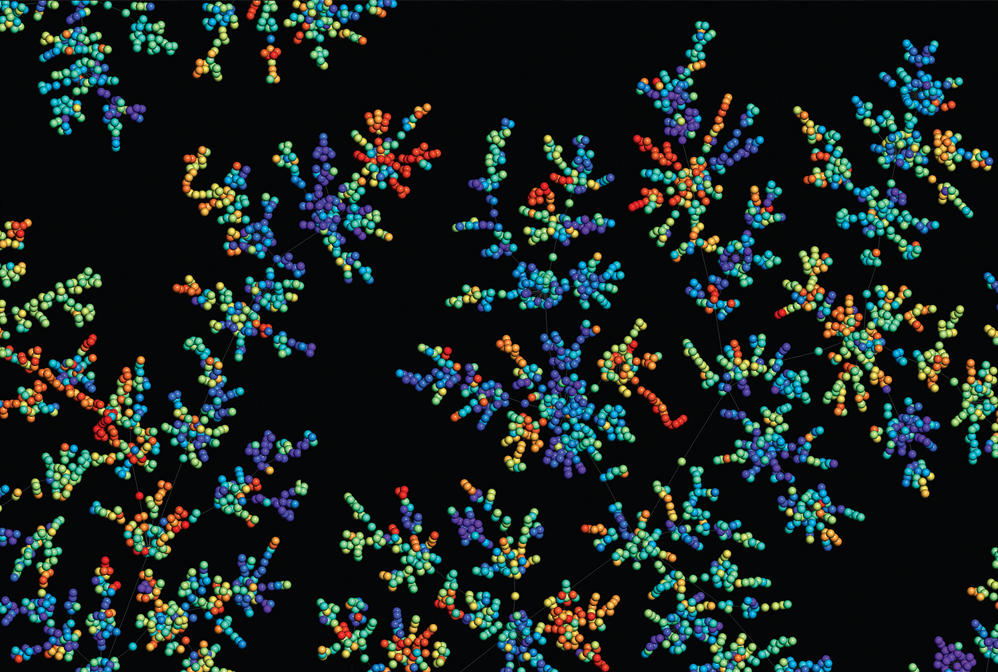
Computer programs help chemists seek out bioactive compounds from among the near-infinite possible chemical compounds.
Credit: Jean-Louis Reymond
Pharmaceutical scientists searching for medicinal chemicals have massive datasets of biological measurements and chemical libraries of potential drug candidates. Computational tools are helping researchers navigate that expansive chemical space. Machines help propose new bioactive compounds, optimize the potency of existing drugs, and repurpose them to treat other conditions. Machine learning algorithms are also helping chemists propose new drugs from scratch. With the aid of digital models, researchers can preview how a candidate molecule might interact with a variety of drug targets. “There is always a computational element in early-phase drug discovery now, and I think this will remain also in the future,” Matthias Rarey, head of the Center for Bioinformatics at the University of Hamburg, told C&EN in June 2022.
Chemical simulations
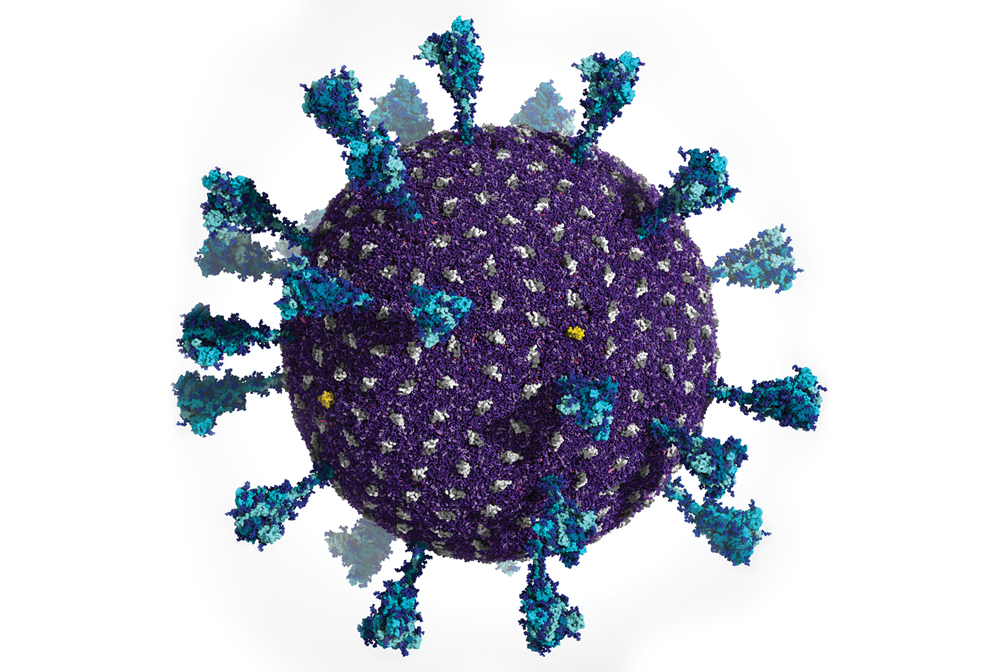
Powerful supercomputers made it possible for researchers to generate this simulation of the Delta variant SARS-CoV-2 viral particle. It contains 305 million atoms that comprise the viral membrane (purple), membrane proteins (gray and yellow), and spike proteins (blue).
Credit: Lorenzo Casalino/Abigail Dommer (Amaro Lab, UC San Diego)
Computers have revolutionized how scientists create models of chemical systems. Just decades ago, computational chemists could simulate snapshots of only a handful of atoms at a time. Today, scientists from a diversity of fields make movies of molecular systems containing millions of atoms at a time. They accomplish this feat with a combination of methods such as density functional theory—a quantum calculation technique that predicts key properties of atomic systems from first principles—and molecular dynamics simulations. These simulations enable scientists to predict molecules’ physical properties in silico. By running complex codes on supercomputers, a team of researchers led by Rommie Amaro at the University of California San Diego and Arvind Ramanathan at Argonne National Laboratory were able to create a molecular representation of the Delta variant SARS-CoV-2 viral particle that comprises 305 million atoms (Int. J. High Perform. Comput. Appl. 2021, DOI: 10.1177/10943420211006452).
Protein design
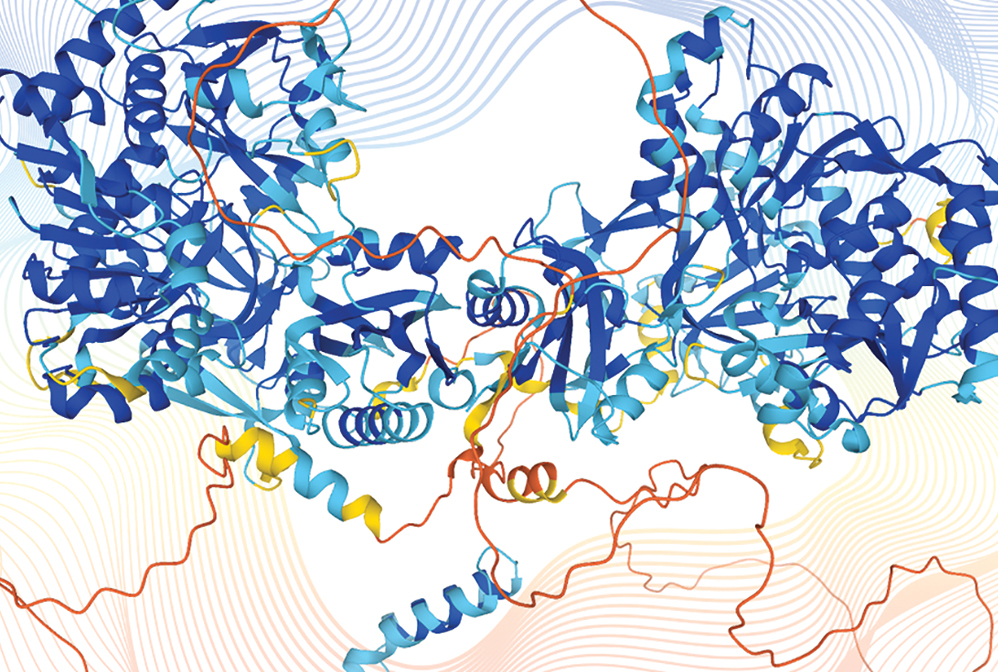
Artificial intelligence programs predict the 3D structures of proteins from genetic sequences (shown here: disco-interacting protein 2 homolog B). AI programs can also propose new sequences to create proteins never before seen in nature.
Credit: Karen Arnott/EMBL-EBI
Almost 70 years ago, the chemical structures of proteins were still a mystery. Yet by the late 1990s, David Baker, now director of the Institute for Protein Design at the University of Washington, and his team had developed a code called Rosetta that could predict folding in an amino acid sequence. This technology helped launch the field of protein design. Chemists can now perform predictive calculations on a massive scale thanks to myriad machine learning–powered programs. For example, in 2021, the Alphabet-owned technology company DeepMind released a public database generated by its machine learning algorithm AlphaFold. The database now contains over 200 million individual proteins from over 10 million species. And chemists are training artificial intelligence–powered algorithms to design proteins from scratch to fit a desired purpose, such as targeted antibody treatments, molecular machines, and catalytic enzymes.
Materials discovery
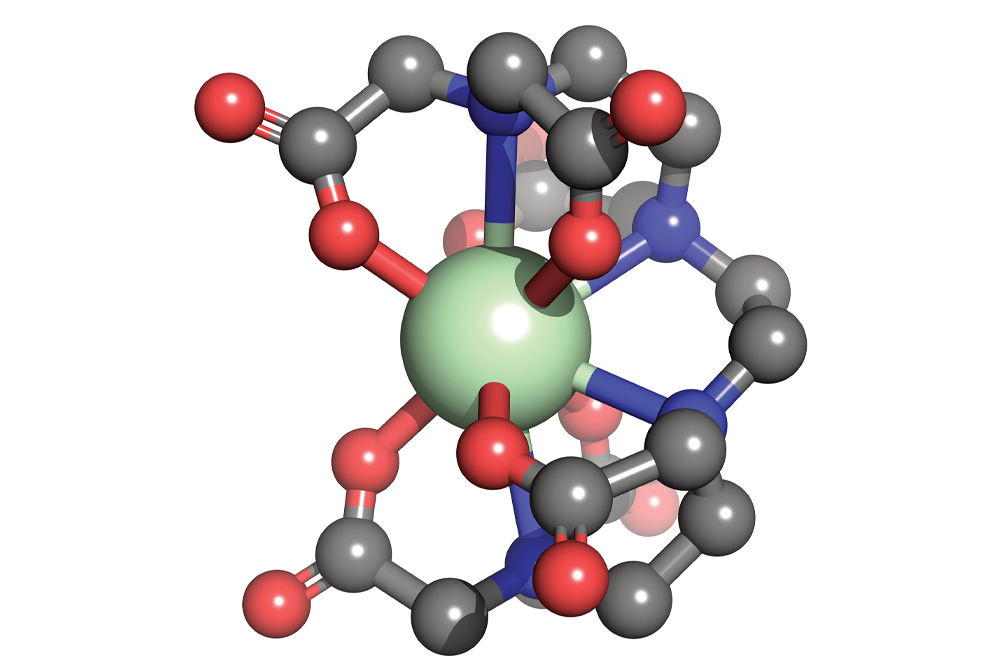
Materials scientists dream up new molecular compounds, such as this organometallic complex composed of a neodymium core (green) bound to an organic ligand at 10 sites (carbon = gray, oxygen = red, and nitrogen = blue).
Credit: Ping Yang/Michael G. Taylor/Los Alamos National Laboratory
Chemists today have an arsenal of synthetic methods and computational tools at their disposal to develop new, bespoke materials. Sophisticated chemical simulations allow scientists to predict molecular materials’ physical properties, such as their ability to absorb gases, store energy, and bond to specific metals. The insights gained from these computations are helping chemists develop innovative technologies, such as new catalysts, more-efficient fuel cells, and molecular components for quantum information devices. Artificial intelligence and machine learning programs continue to gain something akin to chemical intuition, allowing them to propose new materials from prompts in a process called inverse design. The interplay between computational predictions and laboratory synthesis continues to push the boundaries of chemical theory and experiment.
Lab automation
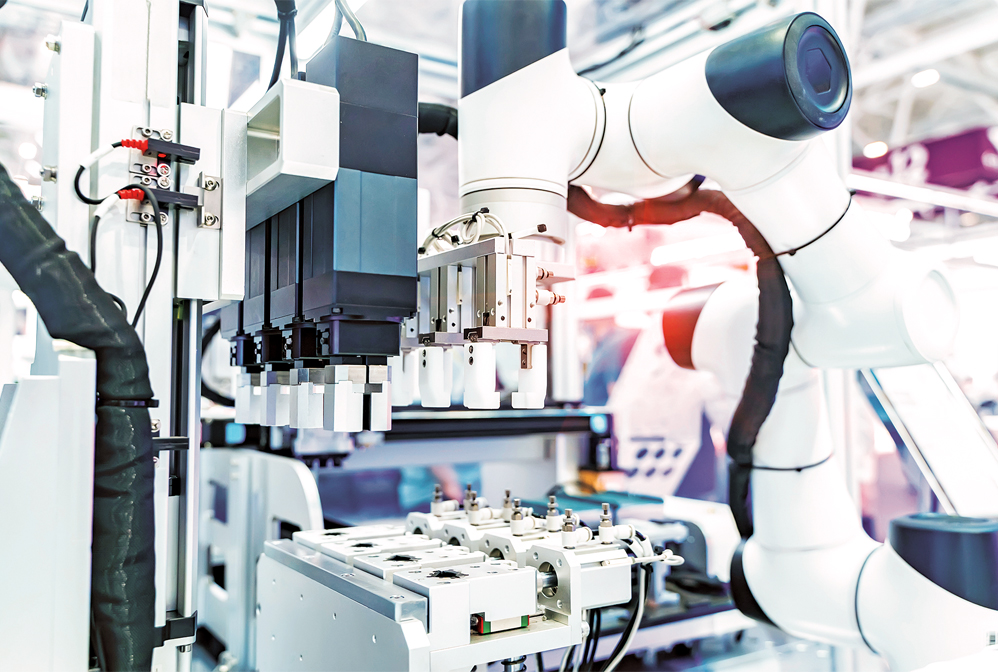
Even common laboratory equipment contains digitized components.
Credit: Shutterstock
The modern chemistry laboratory is packed with computer chips, such as in programmable laboratory equipment and data processing software, that help scientists streamline their experimental workflows. As chemists train artificial intelligence programs to become more chemically savvy, machines are getting better at interpreting data, discovering synthesis protocols, and executing full experiments with little or no human intervention. Researchers in academia and industry are now investing in fully automated cloud laboratories that might someday perform experiments end to end. Users could then access these labs from anywhere in the world. Computational chemists don’t expect these technologies to replace human scientists. “I like to say that artificial intelligence is actually augmented intelligence,” Maxim Ziatdinov, a research scientist at Oak Ridge National Laboratory, told C&EN in February 2023. AI-powered automation processes could make it easier for chemists to tackle more ambitious research projects.
Chemistry communication
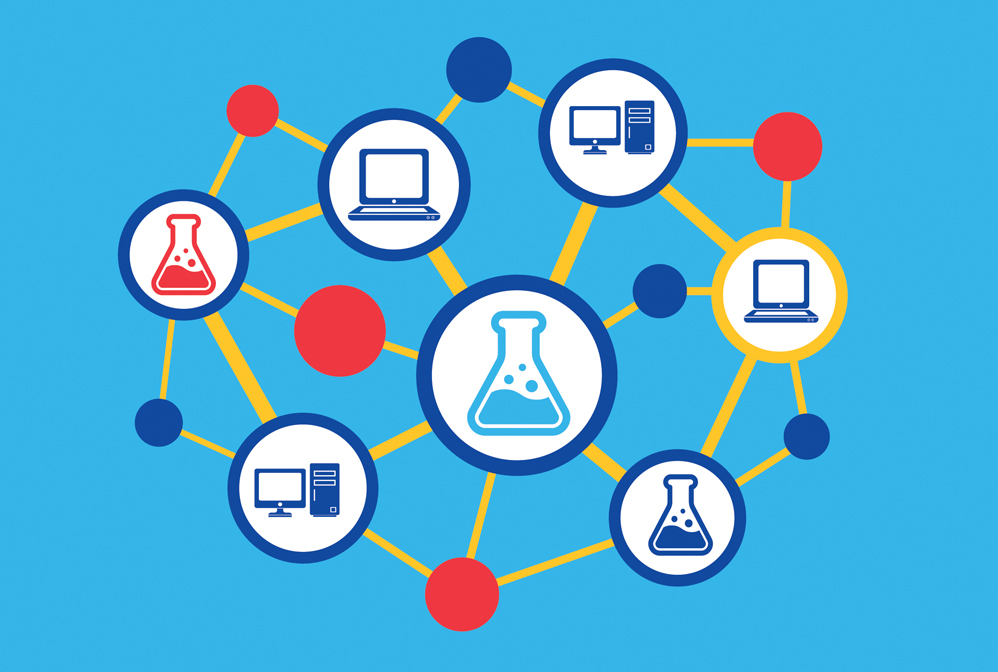
Chemists build communities online to share data, foster collaborations, and discuss discoveries in real time.
Credit: Shutterstock
The internet has fundamentally changed the way chemists communicate with one another and keep abreast of research. It is now common for research collaborations to begin on social media platforms, where chemists discuss breaking discoveries in a public forum. Preprint servers and other data-sharing sites also allow chemists to access publicly available code and data libraries. The preprint server ChemRxiv, which hosts scientific articles before peer review, launched in August 2017. Since then, its users have downloaded content more than 10 million times. Open-access resources were critical for scientists racing to combat the COVID-19 pandemic. “I think what we learned from this pandemic is there is a need for sharing resources and information, and fast collaboration,” chemist Joaquín Barroso-Flores of the National Autonomous University of Mexico told C&EN in July 2021.
Join the conversation
Contact the reporter
Submit a Letter to the Editor for publication
Engage with us on Twitter